Data is the fuel for digital transformation in many industrial and manufacturing industries, where the importance of data-driven decision-making continues to increase. Manufacturing companies continue to search for solutions that can improve process efficiency, reduce unscheduled asset downtime and enhance connected worker capabilities. Still, many clients must learn how to build a scalable and trusted industrial data foundation.
Industrial data often resides in disparate or siloed systems, making it time-consuming and resource-intensive for data scientists and application developers to locate, consolidate and derive meaningful insights that unify knowledge across assets, processes and people.
The industrial data challenges: A multifaceted problem
Industrial data is a goldmine and has the potential to reveal invaluable insights into everything from equipment performance to energy consumption patterns. However, extracting these insights is not an easy task for most enterprises as they commonly face challenges, including:
- Data silos and accessibility: Industrial data is often scattered across a complex network of systems, from legacy equipment to modern sensors. Making it difficult for teams to access the correct data quickly.
- Explosive data growth: The sheer volume of data generated by modern industrial operations is staggering. Existing systems may need help managing and processing this data effectively, slowing down analysis and decision-making.
- Lack of context: Raw data or analysis done on it without any context is meaningless. More than simply collecting data is required. Understanding its relationships to assets, processes and events is crucial for making informed decisions.
- AI's industrial gap: While AI holds immense promise, many current models need more domain-specific knowledge to address complex industrial problems. Scaling AI solutions also requires robust data pipelines and efficient model management.
- Static workflows: Traditional data management approaches often necessitate custom-built applications, limiting the ability of front-line teams to leverage data for real-time decision-making easily.
IT/OT/ET: The strategic solution
A strategic approach is emerging to address challenges: the unification of IT, OT and ET data. This integration enables more informed decision-making and lays the groundwork for the development of customized applications and the adoption of advanced AI solutions.
The goal is not to eliminate the distinctions between these domains but to create a unified strategy that brings together experts, platforms and data from OT, IT, ET and engineering fields. Innovative data and digital platforms facilitate this convergence by merging diverse data types, including audio/visual and unstructured data, making it accessible and valuable to a broader audience within and outside the organization. We have multiple data sources in plants – industrial protocols for PLC/SCADA/DCS/MES/data historians, ERP/CMMS/Service Management, plant documents like P&IDs, engineering, FMEA, etc.
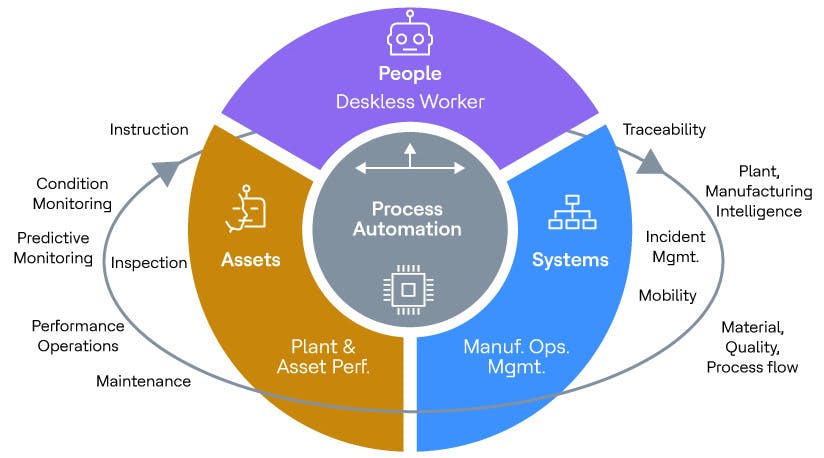
Our partners like SymphonyAI are at the forefront of addressing these challenges. Their IRIS™ platform, a robust industrial data foundation, is designed to facilitate IT, OT and ET data convergence at a petabyte scale. Combining HCLTech’s industry-leading solutions like Real-Time Manufacturing Insights (RMI), Unified Manufacturing Platform (UMP) and an OT-IT convergence suite with the IRIS™ platform, SymphonyAI goes beyond data integration and wrangling. They emphasize the contextualization of data, transforming raw information into meaningful, actionable insights for users across the organization. This comprehensive approach unlocks the hidden value within industrial data. It expands the availability of predictive and generative AI capabilities, enabling industrial organizations to make data-driven decisions and drive operational excellence.
DataOps: Contextualizing and unifying industrial data
Central to the Industrial DataOps strategy is contextualization. By establishing meaningful relationships between different data sources and types, contextualization empowers users to find and leverage relevant data across operations easily. For instance, an engineer might easily interpret sensor data from a pump, but a data scientist might need help with facility-specific naming conventions. Contextualization links the pump's data to its asset hierarchy, work orders and related components and processes, making it understandable for all.
Scaling AI-Powered Insights
To truly maximize the value of industrial data, sophisticated models are needed to derive deep insights. Role-based AI copilots offer the necessary simplicity to interact with these models and obtain actionable information. The combination of advanced models and user-friendly interfaces empowers operators to make optimal decisions confidently.
Industrial DataOps platforms are revolutionizing data management by providing low-code or no-code solutions for application development and model lifecycle management. This democratization of DataOps allows non-expert users to manage data and develop analytics independently, all within defined governance frameworks.
Conclusion
Industrial DataOps is more than a technological advancement - it's a fundamental shift in how industrial and manufacturing sectors approach data. By breaking down data silos, making data usable, contextualizing it and enabling its operationalization, industries are setting the stage for a future where data-driven decision-making is responsible for driving success. This transformation promises increased efficiency, innovation and a competitive edge in the rapidly evolving digital landscape.
Glossary
- AI: Artificial Intelligence
- DataOps: Data Operations
- IT: Information Technology
- OT: Operational Technology
- ET: Engineering Technology