Unifying data landscape for enhanced analytics and collaboration
Our client is one of the world’s largest home furnishings retailers, operating in 60+ countries, offers immersive shopping experiences to their customers enabled through a wide and complex supply chain network consisting of franchisees, suppliers and manufacturers.
In our client’s ecosystem, there were a few challenges in their data management and analytics processes. Various teams were using multiple tools resulting in less collaboration, siloed views of data and inordinate delays to trouble-shoot and fix the issues. The business required greater agility in data consumption and decision-making for dynamic planning and execution scenarios. There was an urgent need to create a centralized data platform where data could be ingested from multiple sources.
Our client embarked on a journey to modernize their data and analytics platform. As part of our client's modernization program, HCLTech facilitated the delivery of various business use cases, focusing particularly on supply chain, through the utilization of Azure Databricks Delta Lakehouse. This not only improved collaboration and data sharing, but also enabled faster and advanced analytics and business intelligence capabilities while ensuring adherence to data security policies.
The Challenge
Streamline analytics to navigate data complexities in retail
Our client’s present ecosystem comprised of multiple applications hosted on different cloud and on-prem environments. Listed below are a few challenges:
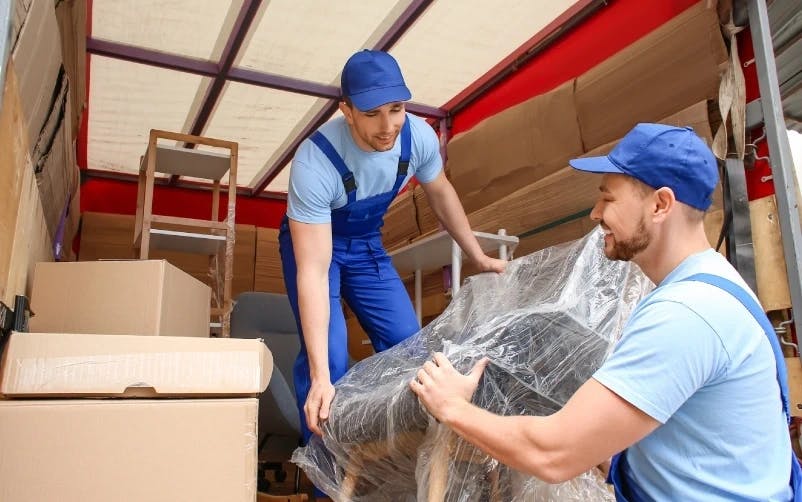
- Lack of advanced analytics and BI capabilities: Our client lacked the necessary tools and infrastructure for advanced analytics and business intelligence, hampering their decision-making process
- Suboptimal cloud architecture: Inconsistent naming, security access management practices in their cloud setup led to inefficiencies and potential security risks
- Disparate data silos: Data was spread across different entities within the organization, leading to fragmented views and hindering collaboration
- Limited data sharing: A complex technical landscape made it difficult to share data across different entities within the organization
- Varying analytics maturity: Different teams had varying levels of analytics maturity, leading to inconsistent support structures and outcomes
- Inefficient data provisioning: Data teams spent excessive time analyzing data locked in multiple systems, resulting in delays in developing insights
- Inconsistent decision making: Fragmented systems prevented users from making informed decisions, leading to inconsistencies in workflows and frequent rework
- Delays in data-driven solutions: Increasing quantity and complexity of information led to delays in prototyping and operationalizing data-driven solutions
- Minimal cross functional collaboration: Lack of collaboration among cross functional teams resulted in inconsistent ways of working and hindered productivity
- Scalability issues: Inability to scale up storage or compute resources due to multiple ETL pipelines hindered the organization’s ability to handle growing data volumes effectively
Azure Databricks Delta Lakehouse was chosen for its status as a top-tier cloud-based data engineering tool which could address our client’s challenges. It enabled the processing, transformation and exploration of big data using machine learning models with data as a product approach.
The Objective
Realizing the benefits of a connected data platform
Our client aimed to cultivate a data-domain-driven culture to enhance analytics ownership and productivity. They sought to leverage a modern data and analytics (D&A) platform to provide data as a service, driving insights and value. Business users were empowered to reuse data products for accuracy, aiding business decisions. Their objective was to establish a single, real-time repository of trusted and organized data of known quality.
Databricks was used with an agile approach to test, learn and generate business value. Users wanted to connect to the data stores and avoid any data migration. By implementing Databricks, they intended to democratize data access, separate storage from compute for infinite scalability, to increase agility, manage costs effectively, eliminate data management challenges without data migration.

The Solution
Transforming data management with a scalable shared D&A platform
The shared D&A platform deployed based on a Data-Mesh approach, instantiated data domains from blueprints, encompassing key capabilities for data value creation such as storage, monitoring, key management, ELT, analytical engines and automation.
Data provisioning of data consumption is categorized into:
- Data Publishers: These were responsible for delivering high-quality, managed datasets that are modelled by business domain entities into a curated layer of the data lake
- Data Products: These leveraged data from data publishers to deliver business results
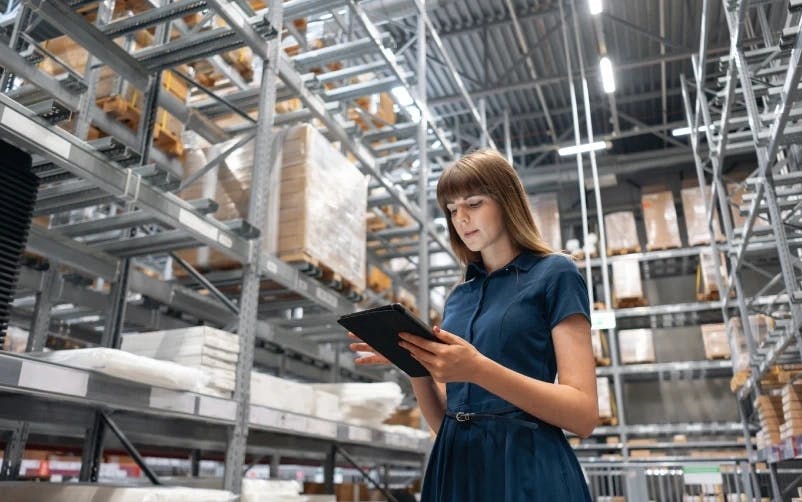
This platform ensured accurate segregation of ownership for each publisher and empowered teams to create interactive, dynamic reports and use Spark’s libraries for data processing, with scalable compute and storage. New ML models and applications could be built on top of Spark, leveraging the integrated workspace in Databricks for collaboration through a multi-user environment, code re-use and simplified workflows. The inbuilt data governance feature of Unity Catalog facilitated centralized access control, auditing, lineage and data discovery capabilities in the enterprise data infrastructure.
The Impact
Utilizing Databricks for scalable integration and optimized data infrastructure
Databricks offers a highly scalable, secure and reliable production environment in the cloud. The platform providing Data as a service (DaaS) through a cloud managed service model, grants clients seamless access to real-time data streams, thereby enabling businesses to operate with greater agility. Users can build, deploy and manage the end-to-end lifecycle of data on the cloud. Teams can perform data exploration and interact with data simultaneously, while independently managing, migrating, testing and handling deployments thereby creating autonomy.
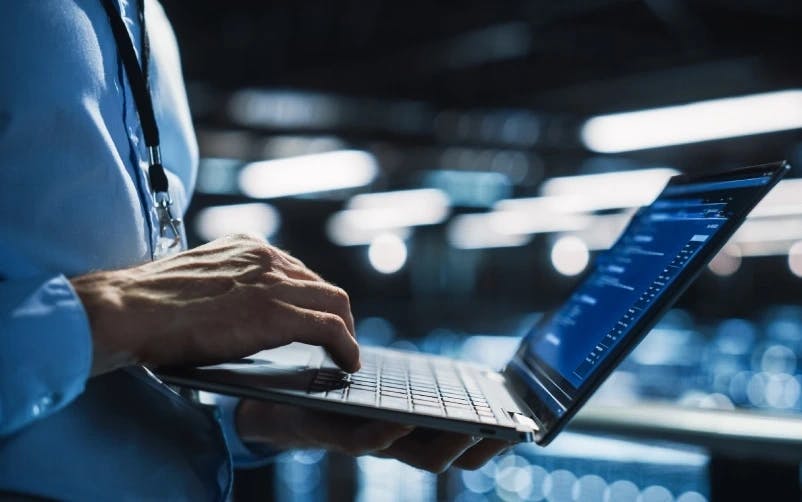
- Faster Analytics
- Faster insights: In Databricks, insights were available 30 times faster than previously. The flattened, nested, structured, unstructured, real-time data allowed the users to analyze it effectively, converting into a relational representation for valuable insights.
- 20x improvement in processing performance using Spark: Databricks significantly reduces batch processing time by handling structured, unstructured, real-time data and ingesting from non-traditional data storages like Oracle, SQL, SAP etc.
- More Secure
- Role based access facilitated by Unity Catalogue: Users have role-based access control, enabling them to directly query the data through an intuitive “simple-to-use” virtual analytics interface. They can access every component of the enterprise data infrastructure, including files, clusters, code, application deployments, caching, dashboards and reports.
- Cost Saving
- 10%-15% increased efficiency and cost savings with a consolidation approach: Increased efficiency leads to direct time and cost savings for large scale tasks.
- Enhanced value delivery with 20%-30% reusable components: HCLTech has developed a portfolio of reusable components and patterns for data ingestion, storage, and consumption which can be re-used across different business units to enhance value delivery.
- Platform Standardization
- Unified data platform: The Data and Analytics platform provides a unified, trusted, and scalable storage solution for all enterprise data.
- Data as a Product: Data is treated as an enterprise asset offered as a service, minimizing duplication, and preparing curated data assets for enterprise-wide usage.
- Realization of Business value
- Key decision making: The data products helped the business users for doing predictive forecasting, demand sensing, logistics management and supply chain management.
- Better governance (through encryption): Data is classified and encrypted according to sensitivity, ensuring secure and appropriate access for all users.