While many of us are still wrapping our head against generative AI, a newbie agentic ai is making waves. And it is more than a buzz word. According to Gartner, by 2028, 33% of enterprise software applications will include agentic AI, up from less than 1% in 2024, enabling 15% of day-to-day work decisions to be made autonomously.
Some may still get the idea that both are one or the same. Before we dive into explaining if they are same or completely different ais, lets first understand what Agentic AI is.
Agentic ai refers to artificial intelligence systems that are capable of autonomous action and decision-making. Now it can be a conundrum that isn’t any AI supposed to do the same. Well yes but the advances in each Ai models are the ones that bring the differentiation. Current models, AI does not act itself, they are simply generating text or visuals when prompted.

But this is going to change with intelligent agents as it meets the gap in agency, Agentic ai use software agents which can achieve goals independently without dependence on human. Agentic can solve complex problems, navigate ambiguity, create plan and make decisions.
Remember last time you asked Chat GPT for or Google Gemini or any other LLM chatbot for some results and got disappointed with generic outputs because current models are restricted till pretrained data and limited by scope and majorly information scrapped off internet. While agentic will not only follow instructions but anticipate your needs and comes up with creative solutions. Let’s understand this by a simple example (figure a) if you ask suggestion for an air purifier maker, while generative ai will pose recommendations but agentic AI can tell you which is favorite among user accounting on real time data and go a beyond, i.e. can also place order for you as well.

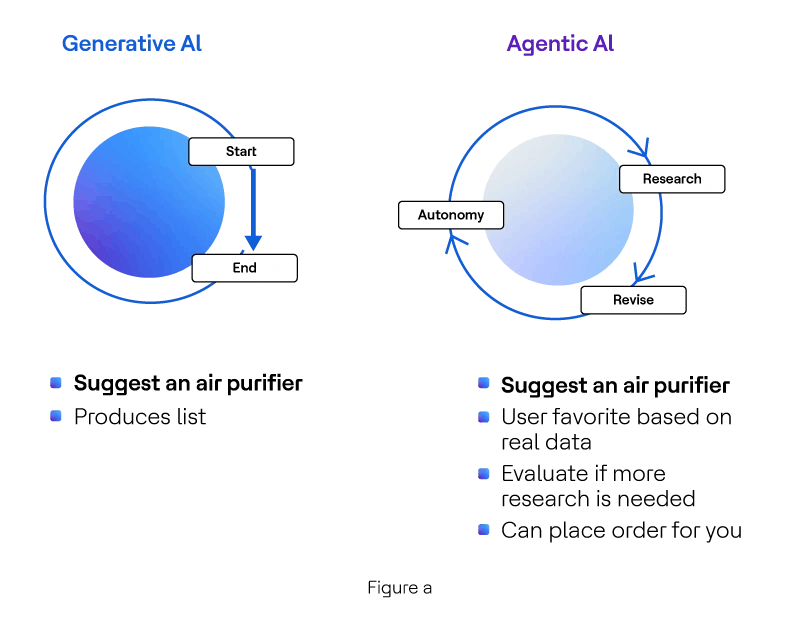
Agentic AI in action – “Different industry use cases”
While GenAi has been the hot topic and disruption in a way, but organizations have been struggling to reap the tangible business value. While Large Language models are striking, but their use cases in enterprises are limited. Large sums of investments being poured into huge LLMs may not yield immediate outcomes, but Agent based AI looks way promising strengthening AI world.
While the primary beneficiary sector is software development, but it does not limit there, we can visualize disrupting transformation in all these sectors.
Software development: Envision AI-driven entities capable not just of creating software but of overseeing the entire development lifecycle. These intelligent agents could autonomously design complex systems, write, debug, and optimize code, as well as manage testing and quality control processes. This shift could dramatically accelerate software creation and overhaul the traditional methods of developing and sustaining digital products. Gartner research shows that by 2028, 90% of enterprise software engineers will use AI code assistants, which is far higher than 14% in early 2024.
IT operations:
- Dynamic problem-solving: Unlike standard AI, which follows set troubleshooting scripts, agentic AI can dynamically adapt to new issues. It integrates data from various IT management systems, learns from previous incidents, and modifies its problem-solving approach autonomously, resulting in faster and more accurate resolution of IT tickets.
- Intelligent actions: Agentic AI can autonomously identify the root cause of issues, isolate affected systems, and implement solutions without human intervention. For example, in the event of a server failure or network disruption, it can reroute traffic or restart servers, significantly speeding up response times and reducing the need for manual monitoring.
- Personalization: For repetitive tasks like password resets and software access, agentic AI delivers a higher degree of personalization. It tailors access permissions and provisioning solutions based on individual user behaviors and patterns, enhancing the overall user experience.
- Predictive approach: By using data analytics, agentic AI can predict when IT systems will fail and perform maintenance before issues arise. This proactive approach helps in optimizing IT infrastructure and maintaining system uptime
Customer service: Agentic AI can fundamentally change the customer service landscape by creating intelligent and tireless virtual agents that manage inquiries and support requests autonomously replacing human support. Queue times could drop by 30-40%, boosting customer satisfaction. These AI-driven systems can analyze customer interactions, predict needs, and provide personalized responses in real-time. From simple FAQs to complex issues—reducing wait times and improving customer satisfaction.
Finance: In the world of finance, AI agents could revolutionize how investments are managed. By continuously analyzing market data, reacting to real-time events, and adjusting portfolios on the fly, these agents could improve trading efficiency and potentially boost investor returns. It just not limits at financial strategies but can help in risk management by scanning through large amount of data to contain exposure and ensure compliance with regulations.
Supply chain: Logistics and supply chain management will see catastrophic changes with agentic ai adoption. Degree of efficiency spun by addressing demand volatility, route optimization, preventive maintenance intensifying operational efficiencies. To top it, can mimic human like decisions to improve customer experience with real time tracking and personalized recommendations.
AI agency maturity
Application of software ai agents do not end here, from cybersecurity to human resources to R&D to medicine, use cases are limitless. Question arises on the adoption which ultimately depends on ai agency maturity. At present, AI agency has not matured, and it requires development.
Majorly there are two systems single agent systems and multi agent systems and that is where the big switch is happening and maturity advances but within journey of even single agent ais to multi agent ais there are various advances across levels, in figure b, you will see three stages in single agent ai itself from basic to dynamic to incorporating ReAct and reflex.


Agent based systems perform function calling to one LLM and handle specific task semiautonomous. With dynamic, it has flexibility to expand tool choices. Similarly incorporating ReAct and reflex single agents self-reflect and correct themselves.
While moving to multi agents from single agents changes the game altogether you have each agent calling to their own LLM unleashing parallel task processing adhering more complex and real-world scenarios. And it goes a notch above with meta included with agents, coordination between agents improves massively and makes MAS highly adaptive to varying environments and probes. Feedback loop with MAS is the highest level of maturity, agents learning from their outputs: agents criticizing, analyzing, suggesting each other gaining higher problem solving.
Trends and predictions: Agentic AI adoption, usage and shift
- In a survey conducted by Research Institute on 1,500 top global executives, 32% of them place AI agents as the top technology trend in data and AI for 2025.
- Agentic AI is expected to contribute between $2.6 trillion and $4.4 trillion annually to global GDP across various industries by 2030.
- In specific sectors, such as energy, investments in agentic AI are expected to triple, from $40 billion in 2023 to over $140 billion by the end of the decade.
Sources:
- https://techinformed.com/2025-informed-the-year-of-agentic-ai/
- Agentic AI – the new frontier in GenAI
While there are risks are posed with its adoption and advancement, agentic AI is the needed fuel for the future to shape business and to speed up autonomous ways of working. We, at HCLTech, are helping business adopt and build the right fit model to cater their needs tied with high level of governance.
To know more, you may write to us at HCBU-PMG@hcltech.com.