Overview
Spotter DQV accelerates data quality monitoring with integrated, customizable, cloud-ready open-source components that scale to the needs of your data platform and use cases.
- Incorporates open-source technologies like Kafka, Spark, HDFS, Yarn, Kibana and Elastic Search
- Supports both real-time streaming data and batch data
- Ensures low latency
- Integrates with metadata and metrics repositories
- Includes data quality monitoring dashboard
Level up trust in data to drive agility, productivity, cost effectiveness and compliance.
See how Spotter DQV improves all data-driven processes
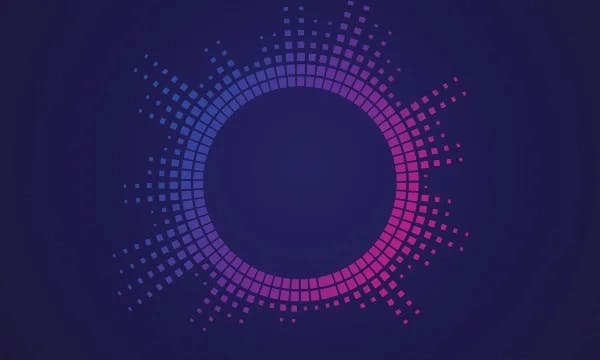
We think these topics might interest you
Spotter DQV Components
The Spotter DQV framework houses a full range of components designed to promote easy and seamless integration into your existing environment and quickly unlock its full benefits.
Source connectors
Kafka and HDFS available in the staging area to enable connecting to both streamed and file-based source data
Metadata repository
Built-in central store of source details, quality check types and attributes and thresholds, as well as a consolidated input for the framework.
Metris Repository
Central metrics store with attribute and total view, as well as support for search and visualizations.
ML/DL models
Machine and deep learning models for outlier detection, data imputation and variance anomaly detection.
Monitoring dashboard
Visualizations of data quality check results, including overall summary and anomaly trend analysis across attributes and dimensions.
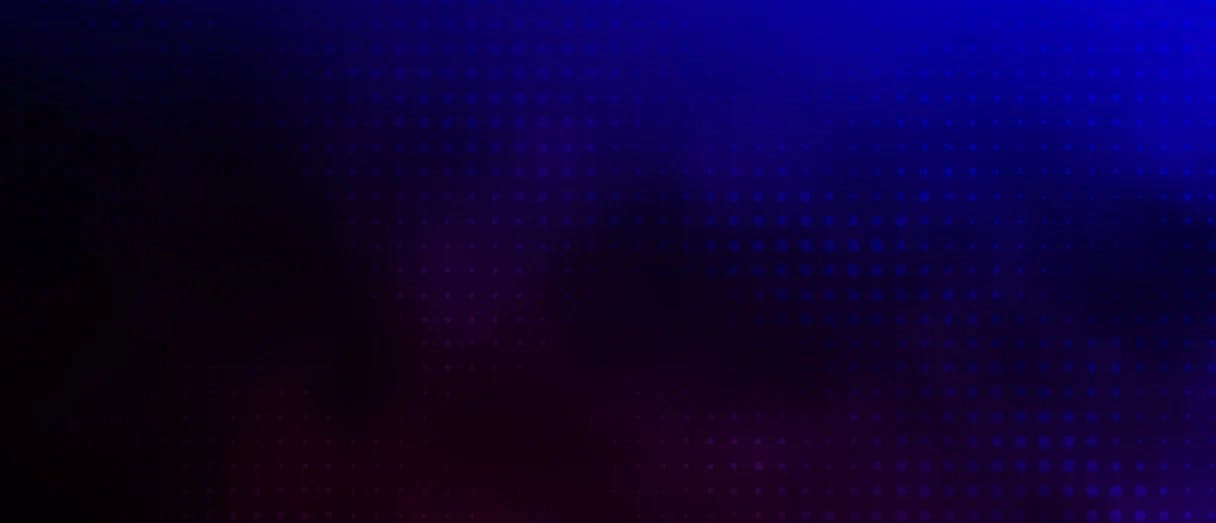
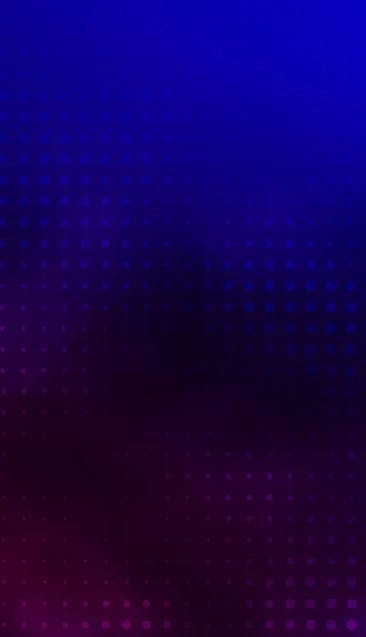
Talk to Our Experts
See how you can empower users with higher-quality data to improve data-driven operations across the enterprise.